
Construction sector is very well known by its poor performance in H&S matters. Data have shown that construction is one of the sectors with the highest accident rate. Beyond this, construction is also one of the sectors that move closely with the economic cycle, boosting fast in period of economic growth and contracting abruptly during recessions. This phenomenon is even more relevant for developing economies, because construction usually accounts for a significant part of the GDP. For these reasons, it is relevant to study and analyze to which extent the survival of a company can be explained by its accident rate as a result of its H&S management, and more, in the construction sector. If a significant relationship exists between those two issues, the proposal is straightforward, in order to prevent economic failure (at least in part) we need to reduce the number of workplace accidents, which in turn will generate both economic and social benefits. In other words, if we can obtain some evidence that lower risk at the workplace produces fewer accidents and this in turn decreases the probability of firm failure, this can be a stronger arguments to persuade practitioners to see effective H&S measures as an investment, not as a cost, with an evident return, firm survival.
Since the Visser’s (1998) “bowtie metaphor”, rivers of ink have run about the interactions between risk and accidents (Hollnagel, 2008). Notwithstanding, this is still an interesting research topic because the non-contingent nature of the relationship among risk and accidents, i.e. not all risky situations ends with an accident and not all safe workplaces are immune to accident occurrence. After a review of this literature, Forteza et al. (2017, p. 62) found that much of these studies are of theoretical nature and claimed that “only a limited number of empirical researches connect risk conditions on site with accidents results”. Using a 6 years panel data of construction sites, these authors found evidence of a positive linear relationship between accident rate of the construction companies and a measure of risk constructed using CONSRAT (Forteza et al. 2016). Despite this evidence, many authors have state that the problem here is that we lack a well stablished theoretical framework connecting risk evaluation models and the causality models of accidents (Khanzode et al., 2012; Swuste et al. 2012). In this paper we develop an empirical study aimed to gain some additional understanding of the complex relationship between risk and accidents. Specifically, we want to test whether or not the level of risk increases the probability of occurrence of an accident in the workplace.
There is also an abundant literature examining the economic impact of health and safety (H&S) in the workplace mostly oriented to estimate its total cost, both for prevention actions and accident occurrence (Breslin et al., 2007; Cheng et al., 2010a,b; Crichton et al., 2011; Feng et al., 2015; Gurcanli et al., 2015; HSE, 2015; Ibarrondo-Dávila et al., 2015; Labelle, 2000; Lim et al., 2011, Reville and Schoeni, 2001; Woock, 2009). Some other studies have found evidence for the association of lower accident rates with higher earnings in firms and more GDP (Barth et al., 2007; Mainardi, 2005). Beyond this partial analysis of the H&S impact in the total benefit equation of a company, some authors have claimed theoretically that H&S is a relevant factor affecting the successful of the management, the economic results and, therefore, the competitive advantage of the firms (Rechenthin, 2004; Teo and Ling, 2006). However, there are very few empirical studies testing these theoretical propositions. One of them is the paper by Argilés-Bosch et al. (2014) who found that labor accidents have a negative influence in economic performance analyzing several sectors of economic activity, construction included. Other example is the recent paper by Forteza et al. (2017) who found that the effect of accident rate on firm financial performance is not linear but quadratic-concave for a sample of construction firms. Interestingly, Forteza et al. (2017) found that Argilés-Bosch at al.’s (2014) hypothesis held for a relatively high interval of accident rate levels, but for a relatively low interval in the levels of accidents rate the relationship between accident rates and firm profitability turned into positive. Although these interesting empirical papers have been welcome, the scarcity of this kind of studies prevent us to obtain a general understanding of the complex relationship between H&S and the competitive advantage of a firm. Scholars agree in the need of more empirical studies on these issues (Argilés-Bosch et al. 2014; Forteza et al. 2017; Rechenthin, 2004).
In sum, in this paper we examine the relationships among the construction site risk, the accident rate, the probability of having accidents and the probability of firm survival in construction industry. In the next section we present the development and statement of our set of hypotheses. Following, we will report the results obtained from our empirical strategy and they will be discussed in detail in the section, and finally we will end this paper with a section devoted to present our conclusion and final remarks.
Hypotheses development
In a recent research, we have offered an empirical test for the general hypothesis that higher risk level in construction sites of a company are associated with more accident rate in that company (Forteza et al., 2017). We found significant evidence of this effect using a panel date from 2004 and 2009. As we have discussed above, we need theoretical models that explain the connections between the available models of risk assessment and the final accidents suffered in the workplace. In order to move a step forward in our empirical understanding of the relationship between occupational risk and accidents at the workplace, we propose here to explore whether or not those construction sites evaluated as riskier also show more probability of having accidents. Therefore our firs hypothesis states as follow:
H1: A higher level of risk in construction sites of a company is associated with higher probability of having accidents.
According to the business strategy literature a company is said to have a competitive advantage if it is able to create more value than its rivals, which is directly related with the firm ability to generate more profits in comparison with its competitors (Besanko et al., 2009). Therefore, by definition the company with more benefits in a specific sector is said to have a competitive advantage. One of the sources for value creation and therefore to build a competitive advantage, is decreasing the total cost of a firm, which is the essence of any strategy of cost leadership. The other source that a company has for creating value is increasing their customers’ willingness to pay for its high quality products or services, i.e. following a differentiation strategy. As we have mentioned above, Rechenthin (2004) has addressed the links between safety in projects and the sustainable competitive advantage of construction firms. This author concluded that an effective safety management can help the companies to achieve a sustainable competitive advantage through a good safety performance. In the literature there is a general agreement that a bad performance in H&S issues, as for example, experiencing a high accident rate, can generate such significant costs that all the firm benefits may vanish, putting in danger its financial stability and even its survival in the medium and long-term. As we have reviewed, the estimation of the total costs of occupational accident has been fully addressed in the literature. Less empirical attention, if any, has been paid to estimate the impact of effective H&S measures on the positive component of the economic benefit equation of a company. It is true that Argilés-Bosch et al. (2014) and Forteza et al. (2017) have provided evidence showing than high accident rates have a negative impact in the economic performance of a firm, but the question of evaluating the economic benefits of effective H&S management systems still remains a an interesting research question.
The construction sector is a relevant economic growth engine that creates a significant volume of employment when the economies are in expansive periods. At the same time is one of the sectors that quickly destroy more employment when the economy turns into a contractive cycle. When this occurs, huge economic and social losses are generated which are extremely harmful for countries and societies. Therefore, in our opinion, any attempt to help construction companies to increase their competitiveness will be welcome and valuable. During recessive periods, it can be seen numerous construction companies stopping their activities and even going bankrupt. Based on the theoretical insights of Rechenthin (2004) and Teo and Ling (2006), as well as on the empirical findings of Argilés-Bosch et al. (2014) and Forteza et al. (2017), we proposed to test empirically whether or not high accidents rates are associated with a higher mortality of construction companies. If this is the case, an effective H&S management can be considered as a strategical tool that can help companies to better survive when they must face bad times. In other words, those resources allocated to create good H&S conditions, which prevent occupational accidents, might be a good investment decision that can make the difference between continuing breathing and passing away. The second hypothesis to be tested in this paper is the following:
H2: A higher level of the accident rate of a construction company is associated with higher probability of company failure.
Empirical design
Our empirical hypotheses are aimed to model the likelihood of two events, the probability of having accidents versus no having in case of hypothesis 1, and the probability failure versus survival of a company in hypothesis 2. As a consequence we have chosen two dichotomous variables as the dependent variables of our two main empirical models. In Table 1 we summarize the names, codes and definition of all dependent and independent variables considered in this paper. Probit and logistic regressions are adequate statistical techniques for modeling the impact of a set of independent variables on a dependent variable that take the values of 0 and 1 (Long, 1997). Since probit and logit yield similar results, the election of which analysis methods to use depends mostly on researcher’s preference and one of the interesting aspects of these techniques is that predicted probabilities can be computed based on the estimation results for given values of the independent variables (UCLA: Statistical Consulting Group, 2017). The analytical formulations derived from our hypotheses can be written as model 1 and models 2 respectively:
For hypothesis 1:
acc_i,t = α + β1 . sri_i,t + β2 . num_w_i,t + β3 . roa_i,t + β4 . sales_i,t + β5 . asetturn_i,t + Ɛi,t (model 1)
For hypothesis 2:
estatei,t = α + β1 . acc_num_w_i,t + β2 . roa_i,t + β3 . sales_i,t + β4 . asetturn_i,t + Ɛi,t (model 2.1)
estate_0_2i,t = α + β1 . acc_num_w_i,t + β2 . roa_i,t + β3 . sales_i,t + β4 . asetturn_i,t + Ɛi (model 2.2)
Dependent variables in model 1 and model 2.2 are dichotomous. The acc_i takes the value 0 if the company did not suffer any accident during the year considered and 1 otherwise. The estate_0_2i takes the value of 0 if the company went bankrupt and 1 if it is operating, both at the end of the period. Differently, the dependent variable in model 2.1., estatei, it is a 3 level rank variable that include an intermediate situation for the firms that falls between bankruptcy and normal activity, which is called the state of “concurso de acreedores”[1] according to Spanish regulations. Depending on how critical the situation is for the company, it may perfectly well continue with its committed activities under this estate, therefore, the firm cannot be classified clearly as a failure or survival case. Therefore, we propose model 2.1. to test for any differentiated impact of the accident rate on these three operation estates.
Table 1. Codes and definitions of variables.
Independent variables
In order to test the hypothesis 1, we need an assessment of the level of risk on the sites in our sample. Several well-structured risk assessment techniques for construction settings are currently available in the literature as for example, QRAM (Pinto, 2014), CHASTE method (Rozenfeld et al., 2010) or TR Index (Laitinen et al., 1999). A detailed description of them is out the scope of this paper, but you can find a good review in Forteza et al. (2016). Even though those methods are really useful and valid, they were narrowly designed to evaluate some specific physical elements that may create/affect risk on site. CONSRAT, the construction site risk assessment tool developed by Forteza et al. (2016) broadens the scope of the risk assessment process because, in addition to the most of those physical elements, it also incorporates several organizational aspects that clearly can affect the risk site conditions. Some of those organizational aspects are organizational structure, complexity, resources or size. Since the accident at the workplace, which one of the variables we want to analyze, is the result of the interactions of how all physical elements are in the site but also of how the site and the company are organized and managed, we have considered more appropriate to use CONSRAT for our empirical study as the tool for measuring the risk on site (for the details of this tool, see Forteza et al., 2016).
Using CONSRAT we have constructed a global risk on site index (RSI) which is based on an exhaustive literature of risk assessment on construction sites. RSI is a composite of ten risk related variables which account for how the H&S plan is fulfilled, which are the condition of order tidiness or access, the level and quality of the protections, the level of fall from height and among other risks related measures that were obtained at site with CONSRAT (see Forteza et al., 2017). RSI (coded as sri_ in model 1) is a continuous variable taking values along the interval 0-1, whit 0 meaning no risk, and 1 extreme risk.
The accident rate (acc_num_w_ in models 2.1 and 2.2) is calculated as the number of accidents suffered by a company divided by the total number of employees of that firm. Obviously, we recognized that the occurrence of either an accident or the company failure can be affected and explained by many other factors beyond the H&S conditions. For this reason, in the three models we add some control variables which are usually considered in the empirical business literature that explains financial and operational/managerial performance (Fairfield and Yohn, 2001;Tan and Wang, 2010). One of these variables is the return on assets (ROA) which is an index of the firm financial performance. ROA is computed as the net income of the firm divided by the average total assets of that firm, and tells you how profitable the set of assets of a company is in generating revenues. In our specifications ROA is represented by the variable roa_i. Another variable usually considered in empirical studies to measure the volume of activity is the revenue obtained by the selling of products or services. Sale volume or revenue is measured in our model by the variable sales_i. Additionally, the variable asset turnover (firm sales volume divided by its total assets) is frequently used as a proxy of organizational efficiency, therefore we introduce this variable (asetturn_i in our expressions) as Argilés-Bosch et al (2014) and Forteza et al. (2017) did.
Sample and data collection
From 2000 to 2010, we visited 957 construction sites of 627 companies in Mallorca (Balearic Islands) to collect information about live conditions using CONSRAT. All sites in our sample were randomly selected. We crossed this initial database with official records of accident rates of construction companies from the Balearic Islands Labor Authority and also with SABI data base that contains financial an economic data of companies. After the matching of the different data sources we ended up with a sample of 502 constructions sites from 272 firms (sample error < 3% at 95% of statistical confidence).
[1] This is the legal situation of a business with serious problems of continuity in which a new CEO is appointed by the Courts with the mandates either of relaunching the firm or dissolving and winding-up the company.
Tables 2.1, 2.2 and 2.3 report the frequency of observations in our sample for each level of the dependent variables and Table 3 shows the sample descriptive statistics for the variables of our models.
Table 4 reports the Pearson correlation matrix for relevant variables of the models we have specified. As it can be seen, both of our binary dependent variables in model 1 and model 2.1 (acc_ and estate, respectively) [1] are significantly correlated with most of the control variables. However, for the hypothesized effects, the event of having accidents (acc_) does not have a significant correlation with SRI (sri_) and the event of survival (estate) does not present a significant correlation with accident rate (acc_num_w_). According to the significant correlations, the event of having accidents in a company is positively correlated with the size and volume of the business. Although, our data do not show a significant positive correlation between risk on site the event of having an accident, we find a significant positive correlation between RSI and the accident rate (0.1260, p < .05), which is line with the idea of our hypothesis 1. Regarding the firm survival event which is measured by estate, the significant correlations also suggest that bigger and more profitable business with less asset turnover are associated with less failure events. Although the correlation between firm survival and the accident rate is not significant and too low, the sign for this relationship is positive suggesting a pairwise behavior against with our hypotheses 2.
We use several empirical strategies for our hypotheses testing that are appropriate for our data set. For model 1 we use two methods, probit regressions and random effects probit regressions for model 1. The difference between them is how the structure of data is considered to fit the model, data are taken as pooled in the former method and as panel data in the later. For model 2.1 we use ordered probit regression which is the appropriate method to fit regression models with discrete dependent variables. Finally, we use probit regressions (pooled) for model 2.2.. We cannot fit the model as panel data due to the low observed with-in variance in the dependent variable for most cases in our sample. The empirical fit of all models has been done using STATA 13.1.
[1] Note than estate_0_2 it is not in included in the correlation matrix because is just subsample the variable estate excluding the cases under the state of insolvency procedure)
Table 5 shows the results of fitting model 1 which is related with hypothesis 1 (the event of having accidents versus not have them in a construction firm depends positively on the level of assessed risk on sites of that firm). Columns (1) and (2) present the baseline models only containing the control variables, while in (3) and (4) we report the results of fitting our complete model 1 using pooled probit and random effects respectively.
For baseline model with pooled estimators (column 1 of Table 5) the results shows a good behavior of the model (LR Chi2(4) = 62.63, p-value < .000). All the estimated parameters are significant. The results suggest that construction firms that are bigger in number of employees, that are more profitable and that has a higher ratio of asset turnover (Sales volume/Total assets of the firm), has more propensity to experience accidents. Although the marginal effect of all independent variables on the probability of having accidents are really small (very small values of the estimated parameters), this may be suggesting that the excessive work load when the volume of activity is very high can be dangerous. However, we do not find an easy explanation for the positive estimated parameter of roa_ (.0033, p-value<.1), beyond the possibility that those firms that are more profitable might stand better to bear the total costs of accidents and behave careless in H&S measures. The negative estimated parameter of sales volume (-1.17e-06**, p-value<.05) even when it is very small, is logical, those construction firms with less revenues from operations may face liquidity constraints and therefor might reduce their investment in H&S measures. When we introduce the explicative variable that measure risk on sites (SRI) to test the hypothesis 1 (see column 3 in Table 5), all estimated parameters (included the one for RSI) are not significant. Additionally, the ability of the model for fitting our data disappear as the Likelihood-ratio test is no significant (LR Chi2(5) = 2.81, p-value=.7293). This result may be consequence of our unbalanced panel data. The small number of observation in specification (3) (306 observations) comes from the few cases with full data after crossing our SRI data with the economic information from SABI data base needed for the remaining variables.
For baseline model with random effects estimators (column 2 of Table 5) the results shows significant goodness of fit (Wald Chi2(4) = 42.71, p < .000). According to this, bigger construction firm with more asset turnover show higher probability of having accidents. However, when we introduce the explicative variable that measures risk on site (sri_) in the specification, see column (4) of Table 5, we did not find any significant effect of risk on sites over the likelihood of having an accident. Despite this, the sign of the estimated parameter is positive as suggested in hypothesis 1, although since the estimator is not significant this is meaningless. Moreover, the Wald test for goodness of fir for our model in column 4 is not significant, what tell us that the model 1 does not fit well our data. As we have suggested above this may be due to our unbalanced panel data. Table 5 also report the likelihood ratio test (LR test) for the significance of the variable rho (proportion of the total variance contributed by the panel-level variance), as it is significant for a p-value<.000 we can confirm that there is enough variability between firms to favor a random-effects probit regression (columns (2) and (4) in Table 5) over a standard ordered probit regression (columns (1) and (3) in Table 5).
Summing up, Table 5 reports all results of fitting model 1 using two estimation methods pooled probit estimators and random effects probit estimation. Despite the sign of the estimated effect of RIS on the event of having accidents is positive as expected, we did not find any significant effect of level of risk on site on the probability of having accidents. Therefore hypothesis 1 cannot be confirmed. One of the explanations for the statistically insignificant effects of RSI on the probability of having accidents we have found, might be the small variability in RSI in our sample, i.e., the bias towards risk that we have found in our sample. This is consistent with the high accident rate inherent in the nature of the construction industry. We observe variability in the accident rate of the sites in our sample, but we do not have enough variability in the levels of RSI of those constructions sites we have assessed, limiting consequently explanatory power of this variable.
The second hypothesis of this paper is aimed to analyze whether or not H&S results effect the survival of a construction firm. We propose to test this hypothesis using two alternative specifications, model 2.1. and model 2.2.. In model 2.1. the dependent variable, estate_ can have three values (0 went bankrupt; 1 went to insolvency procedure; 2 firm alive). We need to fit this model using ordered probit regression. The model 2.2. is the same but excluding the 25 construction firms that went under insolvency procedure during the period analyzed. Table 6 shows the estimations results after fitting our model 2.1. (baseline specification in column (1) and full model in column (2)) and our model 2.2. (baseline specification in column (3) and full model in column (4)).
The overall evaluation of the models is quite good according to the significant test for the goodness of fit of all the specifications, given by the Likelihood-ratio tests which are all significant al the level of .99. Both baseline specifications column (1) and column (3) in Table 6, yield significant parameters estimates for all the control variables included in the models. Therefore, our results support the expected positive impact of profitability (measured by roa_) and volume of sales (sales_) on survival. Although the fitted models gives small estimated parameters in both variables ranging from .0072 to .0080 in case of ROA (see Table 6 columns (2) and (4) respectively) and from 5.79e-07 to 6.56e-07 for sales volume (see Table 6 columns (4) and (2) respectively), all the effects are strongly significant with p-values < .01. Similarly, the variable that is usually used as a proxy of firm efficiency, asset turnover (aseturn_), has a negative estimated effect on the ability of a firm to survive, -.0465 p-value<.1 (model 2.1, column (2) in Table6), and -.0471 with a p-value<.1 (model 2.2., column (4) in Table 6). We do not have a clear explanation for this result as we found a bit counterintuitive. One possible explanation is that the estimated positive impact of asset turnover on the probability of firm death might be reflecting some consequences of the years of economic recession suffered during some part of the period analyzed in this paper, which were especially harmful for the construction industry.
In the hypothesis 2 of this paper we propose that the accident rate of a construction firm (acc_num_w_) has a negative impact on firm survival ability (estate and estate_0_2 variables). According to the results of our fitted models, reported in Table 6, we found evidence supporting this hypothesis and confirming this effect. Both strategies for testing hypothesis 2 have generated similar results. When we regress the three level discrete variable “estate” on our independent set of variables (model 2.1 column (2) in Table 6), the marginal effect of the accident rate on the probability of survival for that firm is negative, -.2542, and significant at 91% of confidence. Similar results from the fitted model when we only consider those alive/dead companies, excluding from the analysis the intermediate estate of being under an insolvency procedure (model 2.2, column (4) of Table6). The estimated marginal effect of the accident rate on the probability of surviving is negative, significant and of a relevant magnitude, -.2644 (p-value<.1).
Therefore, from our data and according to our results, hypothesis 2 is confirmed. When a construction firm operating normally, start to suffering an increment of its accident rate, it can be predicted that its probability of having problems for continuing operating (falling into an insolvency procedure, or worst, going bankrupt) will rise as well. We believe this evidence contributes to the literature devoted to highlight the relevance of an effective and efficient H&S management by reinforcing the message that any effort to put in place effective H&S measures, practices and policies with the objective to reduce the accident rate in the construction industry is indeed an attractive, if not vital, investment.
In this paper we analyze whether risk levels on the construction sites of a company impacts the on the probability of having accidents in that company. Also, we explore whether the accident rate suffered by a construction company is a factor determining the probability of survival of that company. Although these hypotheses can be generalized to any other activity of the economy, the construction sector is an interesting setting for testing them as it one of the sectors with the highest accident rates, with the consequent economic and social losses. As we have reviewed there is not many empirical studies than demonstrate that having a safer firm is a good alternative to help the firm built a competitive advantage. Obviously, closing the business because of financial insolvency is a consequence of losing in the battle camp of competitiveness.
We believe this research provides sound empirical evidence of the potential strategic value of promoting, implementing and maintaining an effective and efficient H&S management in an organization. We have built a good sample of construction firms and sites. We have also applied in a rigorous manner the appropriate methodology for studying and testing our hypotheses. According to our results we did not find any significant effect of the risk on sites, measured with CONSRAT (Forteza et al. 2016), on the probability of having accidents. It is widely exposed in the literature that the relationship between occupational risks and the occurrence of an accident is extremely complex to be modeled because it is ruled under a non-contingency pattern. Our lack of significant results may be explained by this issue or by other empirical reasons as, for example, those related with how we have operationalized our variables or because we considered a turbulent time period or because of the idiosyncratic conditions of the sample considered. We think that much research effort should be address to study the black box of the mentioned non-contingency relation.
Although we did not find support for this first hypothesis, we did find significant evidence supporting the second one. According to our results, we can conclude that there is a significant effect of the accident rate of a construction company on the probability of economic failing. When a construction firm operating normally, start to suffering an increment of its accident rate, it can be predicted that its probability of having problems for continuing operating (falling into an insolvency procedure, or worst, going bankrupt) will rise as well.
Although this paper presents several good contributions, as for example, relevant prediction based on statistical evidence from a data base which is noticeable, it also presents several limitations which open many new interesting trails in the area of H&S research to be explored in the future. For example, some future research questions may consider to address some of the following topics: a) Study how is the process of a changing state from active to bankrupt looking at individual cases; b) Introduce the variable organizational design as a determinant of the probability of having accidents; c) Consider other periods without the years of economic crisis (2008, 2009, etc.) Because is clear that they may have a significant effect on company failure not because H&S issues but for lacking income and bearing an excessive debt; d) Test for multicollinearity among some of our independent and control variables than may generate biased and not reliable estimators; e) Try to obtained other samples that can be more representative of the whole sector, for example, trying to reduce the risk biased of the sites in the sample… and many more.
This research has been partially funded by fund from the Department of Business Economics, the University of the Balearic Islands and the University-Enterprise Foundation of the Balearic Islands.
Argilés-Bosch, J.M., Martí, J., Monllau, T., Garcia-Blandón, J., and Urgell, T. (2014). Empirical analysis of the incidence of accidents in the workplace on firms’ financial performance. Saf. Sci. 70, 123–132.
Barth, A., Winker, R., Ponocny-Seliger, E., and Sögner, L. (2007). Economic growth and the incidence of occupational injuries in Austria. Wien. Klin. Wochenschr. 119 (5–6), 158–163.
Besanko, D., Dranove, D., Shanley, M., and Schaefer, S. (2009). Economics of strategy. John Wiley & Sons.
Breslin, C., Tompa, E., Zhao, R., Amick III, B.A., Pole, J.D., Smith, P. and Hogg-Johnson, S. (2007). Work disability absence among young workers with respect to earnings losses in the following year. Scand. J. Work Environ. Health 33 (3), 192–197.
Crichton, S., Stillman, S. and Hyslop, D. (2011). Returning to work from injury: longitudinal evidence on employment and earnings. Ind. Labor Relat. Rev. 64 (4), 765–785.
Cheng, C.-W., Leu, S.-S., Lin, C.-C., and Fan, C., (2010a). Characteristic analysis of occupational accidents at small construction enterprises. Saf. Sci. 48 (6), 698–707.
Cheng, C.-W., Lin, C.-C., and Leu, S.-S. (2010b). Use of association rules to explore cause–effect relationships in occupational accidents in the Taiwan construction industry. Saf. Sci. 48 (4), 436–444.
Fairfield, P.M., and Yohn, T.L. (2001). Using asset turnover and profit margin to forecast changes in profitability. Rev. Acc. Stud. 6 (4), 371–385.
Feng, Y., Zhang, S., and Wu, P. (2015). Factors influencing workplace accident costs of building projects. Saf. Sci. 72, 97–104.
Forteza, F. J., Carretero-Gómez, J. M., and Sese, A. (2017). Occupational risks, accidents on sites and economic performance of construction firms. Safety Science, 94, 61-76.
Forteza, F. J., Sese, A., and Carretero-Gómez, J. M. (2016). CONSRAT. Construction sites risk assessment tool. Safety science, 89, 338-354.
Gurcanli, G.E., Bilir, S., and Sevim, M. (2015). Activity based risk assessment and safety cost estimation for residential building construction projects. Saf. Sci. 80, 1–12.
HSE (2015). Costs to Britain of workplace fatalities and self-reported injuries and ill health, 2013/14. Retrieved on September 2017 from: http://www.hse.gov.uk/statistics/pdf/cost-tobritain.pdf.
Hollnagel, E. (2008). Risk + barriers = safety? Saf. Sci. 46 (2), 221–229.
Ibarrondo-Dávila, M.P., López-Alonso, M., and Rubio-Gámez, M.C. (2015). Managerial accounting for safety management. The case of a Spanish construction company. Saf. Sci. 79, 116–125.
Khanzode, V.V., Maiti, J., and Ray, P.K. (2012). Occupational injury and accident research: a comprehensive review. Saf. Sci. 50 (5), 1355–1367.
Labelle, J.E. (2000). What do accidents truly cost? - ProQuest. Profess. Safety 45 (4), 38–42.
Laitinen, H., Marjamäki, M., and Päivärinta, K. (1999). The validity of the TR safety observation method on building construction. Accid. Anal. Prev. 31 (5), 463–472.
Lim, S.J., Chung, W.J. and Cho, W.H. (2011). Economic Burden of Injuries in South Korea. Injury Prev. 17 (5), 291–296.
Long, J. S. (1997). Regression Models for Categorical and Limited Dependent Variables. Thousand Oaks, CA: Sage Publications.
Mainardi, S. (2005). Earnings and work accident risk: a panel data analysis on mining. Resour. Policy 30, 156–167.
Pinto, A. (2014). QRAM a Qualitative Occupational Safety Risk Assessment Model for the construction industry that incorporate uncertainties by the use of fuzzy sets. Saf. Sci. 63, 57–76.
Rechenthin, D. (2004). Project safety as a sustainable competitive advantage. J. Safety Res. 35 (3), 297–308.
Reville, R.T. and Schoeni, R.F. (2001). Disability from Injuries at Work: The effects on Earnings and Employment. RAND. Labor and Population Program. Working Paper 01–08. Retrieved on September 2017 from: http://www.rand.org/content/dam/rand/pubs/drafts/2005/DRU2554.pdf.
Rozenfeld, O., Sacks, R., Rosenfeld, Y., and Baum, H. (2010). Construction job safety analysis. Saf. Sci. 48(4), 491–498.
Swuste, P., Frijters, A., and Guldenmund, F. (2012). Is it possible to influence safety in the building sector?: A literature review extending from 1980 until the present. Saf. Sci. 50(5), 1333-1343.
Tan, J., Wang, L., 2010. Flexibility–efficiency tradeoff and performance implications among Chinese SOEs. J. Bus. Res. 63 (4), 356–362.
Teo, E. and Ling, F. (2006). Developing a model to measure the effectiveness of safety management systems of construction sites. Build. Environ. 41 (11), 1584–1592.
UCLA: Statistical Consulting Group (2017). Probit Regression. Retrieved on September 2017 from: http://stats.idre.ucla.edu/stata/dae/probitregression/.
Visser, K. (1998). Developments in HSE Management in Oil and Gas Exploration and Production. Safety Management, The Challenge of Change Pergamon, Amsterdam, pp. 3–66.
Woock, C. (2009). Earnings losses of injured men: reported and unreported injuries. Indust. Rel.: J. Econ. Soc. 48 (4), 610–628.
Papers relacionados

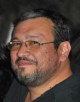



